Error Analysis: The AI Engineer’s Best ROI
Hosted by Hamel Husain and Shreya Shankar
What you'll learn
Learn the most effective technique for improving AI products
Systematically find actionable issues.
Prioritize what matters with data driven approaches.
Why this topic matters
You'll learn from
Hamel Husain
ML Engineer with 20 years of experience.
Hamel Husain is a ML Engineer with over 20 years of experience. He has worked with innovative companies such as Airbnb and GitHub, which included early LLM research used by OpenAI, for code understanding. He has also led and contributed to numerous popular open-source machine-learning tools. Hamel is currently an independent consultant helping companies operationalize Large Language Models (LLMs).
Shreya Shankar
ML Systems Researcher Making AI Evaluation Work in Practice
Shreya Shankar is a PhD student in computer science at UC Berkeley, where she builds systems that help people use AI to work with data effectively. Her research focuses on developing practical tools and frameworks for building reliable ML systems, with recent groundbreaking work on LLM evaluation and data quality. She has published influential papers on evaluating and aligning LLM systems, including "Who Validates the Validators?" which explores how to systematically align LLM evaluations with human preferences.
Prior to her PhD, Shreya worked as an ML engineer in industry and completed her BS and MS in computer science at Stanford. Her work appears in top data management and HCI venues including SIGMOD, VLDB, and UIST. She is currently supported by the NDSEG Fellowship and has collaborated extensively with major tech companies and startups to deploy her research in production environments. Her recent projects like DocETL and SPADE demonstrate her ability to bridge theoretical frameworks with practical implementations that help developers build more reliable AI systems.
Go deeper with a course
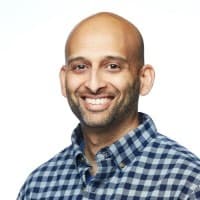
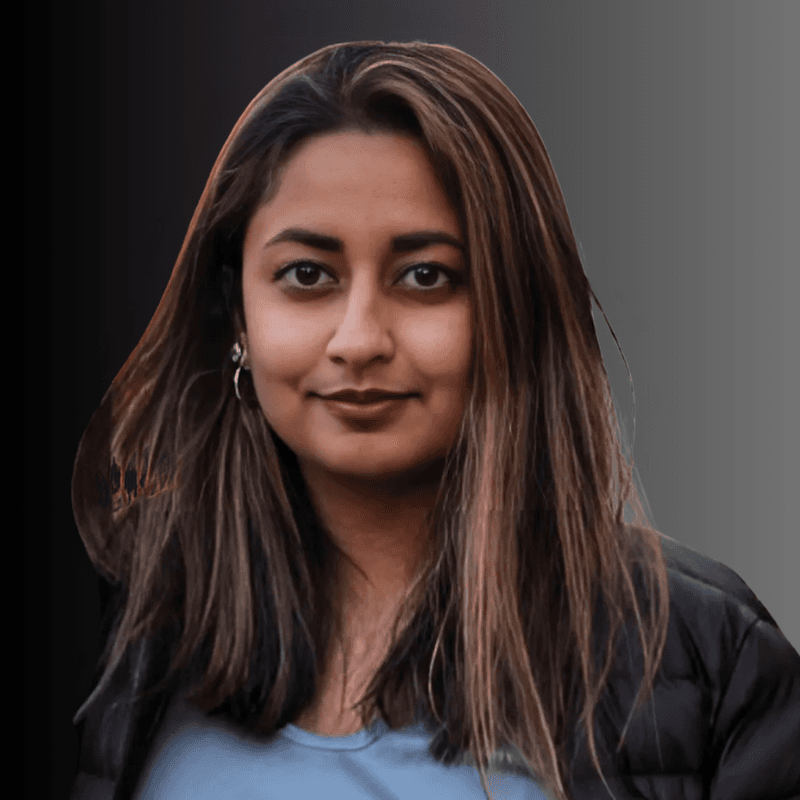
Keep exploring