Data analytics plays a crucial role in modern product management,
helping teams make informed decisions, optimize product performance, and drive growth. This guide will delve into the intersection of product management and data analytics.
The Importance of Data Analytics in Product Management
Data analytics offers valuable insights into user behavior, product performance, and market trends. Here are some key benefits of data analytics for Product Managers:
Evidence-based decision-making: Data-driven insights enable Product Managers to make well-informed choices about product features, prioritization, and resource allocation.
Improved user experience: Understanding user behavior and preferences helps Product Managers tailor products to better address customer needs and elevate the overall user experience.
Success evaluation: Data analytics allows Product Managers to monitor key performance indicators (KPIs) and assess their products' success over time.

Top Practices for Integrating Data Analytics into Product Management
To effectively incorporate data analytics in product management, consider implementing the following best practices:
1. Set Clear Goals and Metrics
Before engaging with data analytics, it is crucial to define explicit goals and metrics that align with your product vision and objectives. Setting Key Performance Indicators (KPIs) and targets for these metrics enables you to evaluate your product's success and make data-driven decisions.
2. Collect and Maintain High-Quality Data
Accurate and high-quality data is the cornerstone of successful data analytics. Ensure you collect data from trustworthy sources, preserve data consistency, and routinely clean your data to eliminate duplicates, inaccuracies, and obsolete information.
3. Choose the Right Analytics Tools
Select analytics tools that correspond with your product goals and your team's abilities. Numerous analytics tools are available, from simple, user-friendly platforms like Google Analytics to more advanced tools such as Mixpanel, Amplitude, or Looker. Opt for a tool that fulfills your requirements and can scale alongside your product and organization.
4. Foster a Data-Driven Culture
Encourage a data-driven mentality across your team by integrating data analytics into your decision-making processes. Promote a culture of curiosity, experimentation, and continuous learning, and empower team members to make data-driven decisions.
5. Visualize and Communicate Data Insights
Data visualization tools can help you comprehend complex data and identify trends, patterns, and anomalies. By illustrating data, you can more effectively communicate insights to your team and stakeholders, enabling better decision-making and alignment.
Examples of Data Analytics in Product Management
Let's explore some real-life examples of organizations that have successfully integrated data analytics into their product management processes.
Spotify: Data-Driven Personalization
Spotify has employed data analytics to create personalized listening experiences for its users. By analyzing user behavior, preferences, and listening habits, Spotify generates custom playlists, recommendations, and even personalized yearly summaries, such as Spotify Wrapped and the new DJ feature. This
data-driven approach to personalization has contributed to Spotify's standing as a leading music streaming platform.
Netflix: Content Strategy Informed by Data
Netflix uses data analytics to inform its content strategy, from identifying popular genres and themes to optimizing artwork and trailers for individual shows. By analyzing user viewing habits, preferences, and engagement metrics, Netflix can make informed decisions about which content to produce, promote, and renew. This data-driven approach has helped Netflix become a dominant player in the streaming industry.
Airbnb: Optimizing User Experience with Data
Airbnb utilizes data analytics to optimize its user experience, from search and booking processes to pricing and review systems. By analyzing user behavior and feedback, Airbnb can identify pain points, iterate on its platform, and create a seamless experience for both hosts and guests. This data-driven approach to user experience has significantly contributed to Airbnb's rapid growth and success.
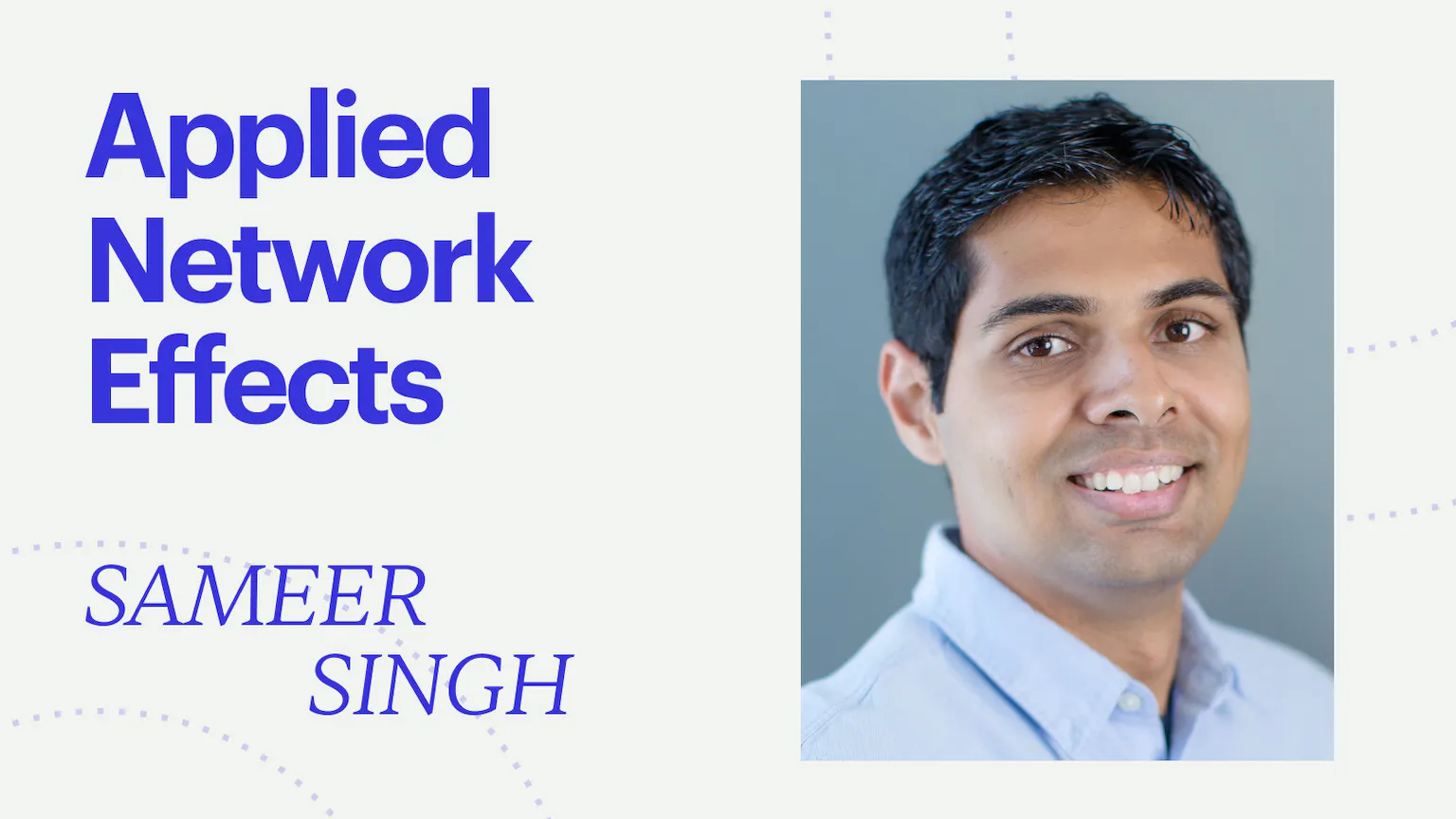
Monitoring and Adapting to User Behavior
In addition to the examples mentioned above, Product Managers should also focus on monitoring and adapting to user behavior. This can be done through A/B testing, which allows you to experiment with different product features, designs, or messaging to determine the most effective approach for your users. By gathering and analyzing data from A/B tests, Product Managers can identify trends, make data-driven decisions, and continually refine their products to better serve users.
A/B Testing and Its Impact on Product Management
A/B testing is a powerful method for gathering quantitative data on user preferences and behavior. It involves creating two or more variations of a product feature, design, or messaging and presenting them to users in a controlled environment. By measuring user engagement and performance metrics, Product Managers can determine which variation performs better and optimize their products accordingly.
Segmentation and Personalization
Another key aspect of data analytics in product management is segmentation and personalization. By analyzing user data, Product Managers can segment their audience based on various criteria such as demographics, behavior, or preferences. This segmentation allows for personalized experiences, which cater to the unique needs of each segment, ultimately leading to higher user satisfaction and engagement.
For example, Amazon uses data analytics to segment its users and provide personalized product recommendations based on browsing and purchase history. This personalization has been a significant factor in Amazon's ability to maintain customer loyalty and drive repeat business.
Balancing Quantitative and Qualitative Data
While quantitative data offers valuable insights, it is essential not to overlook the importance of qualitative data in product management. Qualitative data, such as user feedback and interviews, can provide a deeper understanding of user needs, motivations, and pain points. By combining quantitative and qualitative data, Product Managers can gain a comprehensive view of their users and make more informed decisions.
For instance, Slack, a popular team collaboration platform, combines quantitative data from user analytics with qualitative data from user interviews and feedback to continually improve its product and user experience.
Essential Takeaways for Product Managers
To effectively employ data analytics in product management, keep the following key takeaways in mind:
Set clear goals and metrics that align with your product vision and objectives.
Collect and maintain high-quality data to ensure accurate and reliable insights.
Choose appropriate analytics tools that meet your needs and can scale with your product and organization.
Foster a data-driven culture within your team and organization, encouraging curiosity, experimentation, and continuous learning.
Visualize and communicate data insights to improve decision-making and alignment across your team and stakeholders.
Employ A/B testing to optimize product features and user experience.
Leverage segmentation and personalization to cater to unique user needs and preferences.
Balance quantitative and qualitative data for a comprehensive understanding of your users.
By adopting data analytics best practices, Product Managers can make well-informed decisions, optimize product performance, and drive growth in today's competitive environment.